Learning Complex 3D Human Self-Contact
Mihai Fieraru, Mihai Zanfir, Elisabeta Oneata, Alin-Ionut Popa, Vlad Olaru, Cristian Sminchisescu
Abstract
Monocular estimation of three dimensional human self-contact is fundamental for detailed scene analysis including body language understanding and behaviour modeling. Existing 3d reconstruction methods do not focus on body regions in self-contact and consequently recover configurations that are either far from each other or self-intersecting, when they should just touch. This leads to perceptually incorrect estimates and limits impact in those very fine-grained analysis domains where detailed 3d models are expected to play an important role. To address such challenges we detect self-contact and design 3d losses to explicitly enforce it. Specifically, we develop a model for Self-Contact Prediction (SCP), that estimates the body surface signature of self-contact, leveraging the localization of self-contact in the image, during both training and inference. We collect two large datasets to support learning and evaluation: (1) HumanSC3D, an accurate 3d motion capture repository containing 1,032 sequences with 5,058 contact events and 1,246,487 ground truth 3d poses synchronized with images collected from multiple views, and (2) FlickrSC3D, a repository of 3,969 images, containing 25,297 surface-to-surface correspondences with annotated image spatial support. We also illustrate how more expressive 3d reconstructions can be recovered under self-contact signature constraints and present monocular detection of face-touch as one of the multiple applications made possible by more accurate self-contact models.
Paper
Citation
@inproceedings{fieraru2021learning,
title={Learning complex 3d human self-contact},
author={Fieraru, Mihai and Zanfir, Mihai and Oneata, Elisabeta and Popa, Alin-Ionut and Olaru, Vlad and Sminchisescu, Cristian},
booktitle={Proceedings of the AAAI Conference on Artificial Intelligence},
volume={35},
number={2},
pages={1343--1351},
year={2021}
}
Related Datasets
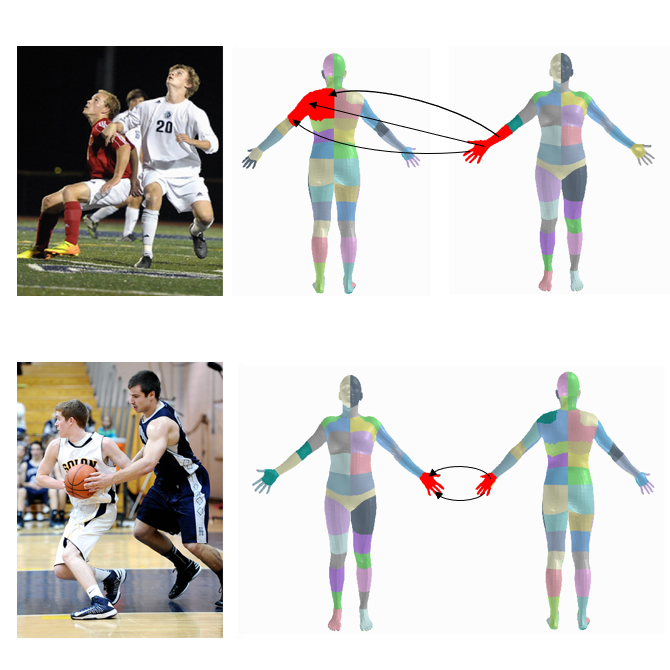
FlickrCI3D
Interaction Contact Signatures: 11,770 images; 14,866 contact events; 138,213 selected contact regions; 81,233 facet-level surface correspondences;
Interaction Contact Classification: 90,167 pairs of people;